table of contents
This post navigates the theoretical fundamentals of AI animation and is meant to be approachable to most people, describing technical aspects in simplified terms. For deeper cuts into technical details and applications in practice, seek level 2 and 3 posts.
The term "frame-by-frame" is thrown around often in this blog, which I've explained so far only as little as necessary. In this essay I will face it head on and analyze its implications in the context of generative AI animation, and describe how it enables what I call "frame-by-frame dream engines" - one of the most prominent aesthetic apparatus of AI video art so far.
Defining "frame-by-frame" (FBF)

One could argue that all moving image, that is somehow captured, is always
divided into frames, played in frames-per-second, and therefore
"frame-by-frame".
In our context here more specifically,
"frame-by-frame" (FBF) means the following:
-
The process of dividing and treating moving image (movies) as a sequence of discrete
stop-moments in time (stop-motion), and actively engaging with the nature of
illusion that emerges from stitching these stop-moments together. In other
words, acknowledging that all of video media is dependent on this principle,
and playing with it for artistic intent, usually in animation, and often deliberately with lower frame
rate to amplify desired illusionary effects.
- An approach to animation using generative image AI, where the AI model itself has no temporal comprehension or sense of continuity, and is generating discrete static frames in isolation. In an such approach, it is up to the artist and additional tools to weave animated imagery from it. This is in contrast to generative video AI, where the generation process inherently works in a temporal domain, taking into account a specific context window of time, and therefore having its own agency in the temporal domain.
For an analogy, think of AI language models. They may generate text word-by-word, but the underlying neural network is processing entire sentences and paragraphs, thus creating continuity of logic and larger structure. That is equivalent to a generative video model that processes sequences of video frames in its neural network. So, a generative image model producing video or animation FBF is like that language model only being able to read and remember one word at a time, always working in isolation from the rest of the potential temporal context.
So in this context, FBF basically defines a subset of
moving visual AI art techniques, and it's the one that's most aligned with the tradition of animation as an art
form and medium. However, "AI art" in general is hardly only a tool or genre of digital
art now, and has become a strange medium of its own...
Dream engines - embracing the strange
Majority of criticism for generative AI output fall into two groups, which
both stem from a stiff belief that gen AI should only be about simulating
existing art and reality: it's either
bad at it and produces objectively lifeless and generic results, or it becomes so good that it
flips our entire creative economy on its head. The latter is more of a "job replacement engine", and if that was the whole
story, there would be far less for the arts to dig into and break new ground
today.
Washed Out "The Hardest Part"
— Paul Trillo (@paultrillo) May 3, 2024
I leaned into the hallucinations, the strange details, the dream-like logic of movement, the distorted mirror of memories, the surreal qualities unique to Sora / AI that differentiate it from reality. Embrace the strange. pic.twitter.com/AlhsVTO78B
Instead here I want to talk about "dream engines": flawed, neural,
hallucinatory machines. AI models built on deep learning, that either haven't
yet reached their intended utilitarian vision, are not meant to reach it, or
are intentionally handicapped and misused. This idea emerges from treating
generative AI as "a new medium with its own distinct affordances", as argued in the meta analysis "Art and the science of generative AI". Its
authors highlight angles to this medium across various fields, however the
notion of a "dream" best describes the potential hallucinatory and uncanny
characteristics of it in terms of aesthetics, which are at the center point of
my analysis here and
personal creative interest.
Such aesthetic characteristics come from the underlying material or "fabric" of the medium, that all mediums have in their own unique way. This fabric, along with its artifacts, can get exposed the more imperfect, unfinished, and unconcerned with representation the use of medium is. In painting for example, this may be the abstract strokes of thick paint on a canvas, as opposed to painstaking realism and depictions of narrative. In deep learning based image AI, the fundamental medium is not just that of digital image, but also the underlying neural network mechanisms that produce these images. It may hold a promise of perfectly simulating its training data and design intent, but when it doesn't, we see past the illusion, into the medium itself.

From left to right: Michelangelo - "David" and "Awakening slave". Van Eyck - "Arnolfini Portrait", Jackson Pollock - "Full Fathom Five" (detail). heladerodeltiempo - "Genesis", Ge:mini - "Day239 wrapped memory". "Toy story 4" (Pixar), Nikita Diakur - "Carousel". Various generative AI images, namely STR4NGETHING - "ST4TEN S1LENC3", untitled image by NowhereManGoes on CivitAI, and finally output from google's "Deep dream".
Until recently, you wouldn't even have the idea to distinguish between perfect and imperfect generative AI. To the general public, it was all akin to toys and games of uncanny creations, or was limited to very specialized use cases, but the research was fast paced, and is only faster today. AI generated content is rapidly mixing in with the rest of our media, the promise of AI detectors is brittle, and fabrication of specific artist styles through AI without their consent is a genuine concern.
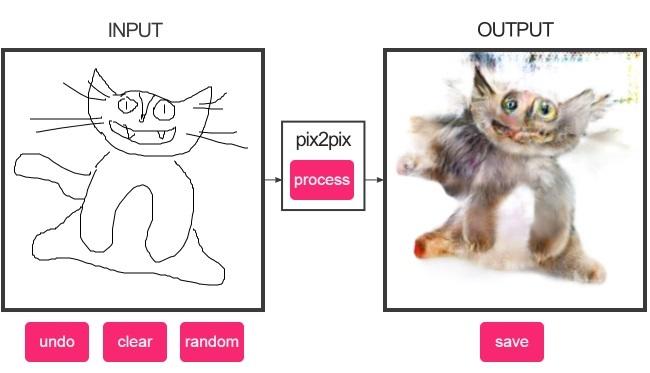
A "dream engine" then to me is an AI tool or interpretation of it that makes no effort to conceal the imperfect and often uncanny neural nature of its medium, which in a way is leaning into Clement Greenberg's favored idea of "medium specificity". I believe this is exactly what Paul Trillo is echoing in his commentary using state of the art generative video AI "Sora" (figure seen above). "Embrace the strange" is about embracing the strangeness of this medium, and not only it can be embraced, but also deliberately used as an instrument. My dear friend and colleague Lukas Winter describes this as "opaqueness" of a medium or technique in his thesis "How Technique Shapes Narrative":
'Transparent technique' entices the viewer to perceive diegetic elements only and forget about technique, whereas 'opaque technique' makes diegetic and technical aspects meet.
- Lukas Winter
Lukas goes on to list many convincing cases of such opaque techniques in
animated short films, where narrative and meaning are intertwined with the use
of a particular, deliberately chosen and strategically executed animation
technique. Naturally I am curious, what ways can there be to use generative
dreamy AI in storytelling that follows similar patterns of opaqueness? Using
AI not because we can, or because it's faster, but because it's the best way
for something particular to be expressed...
Coming back to FBF mode of operating generative AI for animation, it is tempting to
think that discussing such a technique is shortsighted in the grand picture of
today's AI advancements. However, it is particularly the FBF approach that
allows a way to peer deeper into this medium and use it in a compelling
"opaque" way with creative intent in animation, by performing a kind of
mise en abyme
with the medium itself.
The broken telephone of images using Stable Diffusion
The aforementioned "prominent aesthetic apparatus" of AI video art - that of flickering, constantly morphing image, is popularized today thanks to widespread adoption of Stable Diffusion (SD), and how easy it is to set up FBF animations with it. To visualize and understand the core aspect of such animations, let's look at the game of "Drawception":

Drawception is an online browser game that essentially plays like a broken telephone of drawings and their descriptions. It's one of many with such a concept. Each step in a round of this game is completed by a different person, who is only presented with the last drawing or description of a drawing. They then either draw that description, or describe that drawing, continuing the chain of broken communication and loss in translation.
Why are these games so fun? I assume we enjoy the emergent, unexpected twists and turns of miscommunication, when it's isolated within the realms of games and art.
What if the entire round of such broken telephone was played by the same person? Somebody unable to form new memories, forced to read into their own creations over and over, regurgitating it from the same frame of mind. Would such a game retain more of the meaning and content throughout the drawings? Would it get stuck in a loop of infinite repeating interpretations?
Well, that's pretty much what Stable Diffusion is doing whenever it's forced
to "dream" through feedback loops of reading and generating from its own
images.
Dreaming in a frame-by-frame feedback loop
Here is a simplified scheme for the "engine" of SD feedback animations, meant to visualize and distill the most essential steps of it.

Let's say we start by supplying our own starting image of a cat:
- The image is encoded into latent representation by the variational auto encoder (VAE).
- The latent representation gets partially ruined by adding "η" amount of noise to it.
- The denoiser neural network attempts to guess an image (latent representation of it) obfuscated by "η" amount of noise, conditioned by a prompt ("a pet" in this case) and optionally other forms of conditioning.
- The resulting latent representation is decoded into a pixel image that we see and add to our animation.
This is a recursive use of "image-to-image", where the SD model generates a new image more or less loosely based on an input image, and the operation is repeated in what essentially is a solo broken telephone game of images, running in a feedback loop. This ends up creating a kind of self perpetuating hallucination device, repeatedly reinterpretating its own images. Depending on the conditioning, it may be more o less guided to stick to specific content and form. A prompt such as "a pet" allowed it to freely drift across the rather ambiguous meaning of the word.
Here are the key takeaways from this:
- The telephone is broken here due to deliberate introduction of information noise, which neural network then tries to eliminate out of each image by making guesses and interpretations. Noise amount is specified by us.
- The noise and all processing is dealing with encoded, latent representation of images, rather than conventional pixel images. This representation is closer to a distilled meaning of an image, and is somewhat similar to describing an image in a game of broken telephone, instead of just showing the obfuscated image itself. The noise that is added can be thought of as the amount of uncertainty that then results in loss in translation.
- The depiction of the denoiser neural network as a chunk of brain is not coincidental. It's the main component of any diffusion model that holds the "memories" of its training data, along with the artificial "intelligence" that emerges. I like to think of it as a visual cortex that's been separated, yet still filled with biases and "neural" flaws in its perception just like living brains are.
- Other than parameter manipulation and outside conditioning, the denoiser "brain" is static, doesn't learn, doesn't form new memories, and works on each animation frame in isolation from the rest. In other words, it has no sense of continuity and time.
This kind of feedback loop is not a necessity to create animations using SD, but I'd argue it's the most interesting and extensive way of doing it, giving birth to an entire sub-genre of AI art, and leading to the morphing aesthetics that to many people are synonymous with AI animation as a whole.
In practice though, when such a feedback loop is employed, it is only the first step in countless potential techniques and experimentation. In tools such as Deforum, It is usually supported by additional steps of processing and guidance that happens on the image itself (after each 4th step in the loop). Common workflows include stylization of video material, or making the now stereotypical infinite zoom animations.
Having explained this essential element of current day FBF AI animation, I
must come back to my earlier contemplation. Why and when to make use of it?
For most people and cases, "fun" is enough of an answer, especially when it's
the most malleable and susceptible to DIY tinkering thanks to SD being open
source. However, having spent the majority of my thinking time on it for almost a
year now, I owe it to myself and to you to dig for deeper answers.
Emergent behavior of a feedback loop
Here I would like to introduce another useful analogy - that of media feedback setups in general:

From James Crutchfield's paper "Space-time dynamics in video feedback", discovered and made available online thanks to resources on "Softology"
The most straightforward and intuitive kind of feedback system is that of a camera looking into a screen, that displays that camera's output. Today, I'm sure most have encountered this in their zoom calls when somebody points their webcam or phone to the screen.
If it didn't already "click" for you, I hope it does now, that the "dream" feedback loops of SD is a lot like this traditional video (or audio) feedback, except that it has a way denser layer of processing involved. Luckily, being now an old phenomena in relative terms, video feedback is well explored and, I'd argue, can help analyze the SD feedback by drawing parallels.
Video Feedback is a great example of real-time evolving self-organising systems near the edge of chaos. With a relatively simple camera-monitor/TV set-up you can produce a vast spectrum of time-based fractal species, many of which bare great similarity to the complex dynamical systems found in nature.
- Paul Prudence (on his website "Dataisnature")
There is no "brain" in traditional video feedback, and no computing, yet it
can appear very complex through emergent behavior that comes from noise and
simple alterations in its recursive signal: encoding in the camera, decoding
in the video receiver, displaying it on the screen, capturing it through the
camera lens, and especially deliberate disturbance and transformation
introduced into the loop. The artist seen in the figure above, and many
others, have taken advantage of this to open up its emergent, fractal
dynamics.
I argue that the very basic SD feedback loop by itself is equivalent to the typical endless corridor that you get when pointing a webcam into a zoom call, which is to say, that it is only scratching the surface of what may potentially be possible when such feedback process is sufficiently provoked. With SD, typical transformations of scale, translation and rotation employed in video feedback usually don't lead to complex behavior, because its inherent need to make sense of, and produce coherent pictures overpowers subtle alterations. Such transformations are commonly and intuitively used to produce moving image, scenes, and change of composition in SD animation, but the most interesting properties would be that which are the least possible to reliably predict.



I will humbly admit that I too have only scratched the surface of this so far, and for the most part have treated the few unexpected behaviors I encountered as "happy accidents" and glitches. The intuitive modes of operation by using source video, moving camera, changing prompts to produce morphing content, and others, are fun enough to keep using these FBF dream engines as yet another tool in our arsenal. However, let video feedback be a lesson, that taking something usually seen as an inconvenience of a medium, and letting it be the content, the central point of artistic exploration, can lead to fascinating results. The emergence and exploration of video feedback as a genre is a story of a medium looping onto itself, with nothing to show, but only itself.
In the case of Stable Diffusion (V1.5), during feedback animation, this "medium"
that it is looking back into, is its own enormous
neural network of 860 million parameters, through which images get pulled through over and over. Then, if you dare
to image this network as a kind of artificial chunk of brain, like I do, then
perhaps a deeper way to explore this medium is to treat it as AI
psychonautics, looking for the hidden and strange in it, and perhaps even
allowing it to teach us something about our own human perception and its
biases.
Implementations in practice (bonus)
Lastly, as a bonus I will briefly overview some of these FBF dream engines in the wild. A detailed technical breakdown of FBF implementation is best reserved for a separate article, and a guide for all of AI animation can be found in my article on it, which also includes a category specifically for FBF techniques.
If you're reading this, most likely you have been exposed to tools that enable SD feedback loops. Generally, FBF dream engines are either in the form of streamlined app services, or extensive and complex toolkits. This is not to say that it has to be complex in an overwhelming way, or in a "you don't need to understand it" way. In most customizable Stable Diffusion implementations, such as A1111 WebuUI or ComfyUI, the feedback loops may be initiated through a simple function commonly called "loopback". That is enough to get you started in the spirit of the FBF feedback loop scheme that I have presented.
streamlined apps (blackboxes) | extensive hands-on toolkits |
---|---|
|
|
The special sauce in the most advanced tools is the ability to meticulously schedule and orchestrate countless parameters and conditioning, however, as mentioned before, the most important recursive processing happens through traditional image operations (as opposed to relying on some sort of AI models). Each loop the images may be scaled, rotated, brightened, darkened, sharpened, blended with your input video, etc. In fact, a big part of what these toolkits offer is actually catered to transformative video workflows, which I have left out on purpose in this essay, because mixing in real video footage into these dream loops causes them to be grounded and rather stuck on rails. This is not necessarily a bad thing, it all depends on one's goals, but I am becoming increasingly convinced that it's an artistic dead end when it comes to AI art, especially when video models are promising a better version of the same thing.
Cadence, "thorough" and "non-thorough" FBF loops

In some cases, the animation frames are generated through SD in "cadence" (as called in Deforum),
with intentional "gap" frames. I would call this a "non-thorough" FBF
approach. This is particularly meaningful when certain image operations are
carried out on all frames (including "gap" frames), which produces smoother,
continuous change and motion, while the SD generated textures are cross-faded.
To animators, the most obvious analogy would be the use of "key" frames and
"in-between" interpolation through animation software.
Real-time and live implementations
With increasing SD back-end and hardware specific optimizations, FBF techniques are more and more often employed in real time applications. The most blunt way to achieve real-time interactivity, is to render each frame fast enough to accumulate sufficient frame-rate (20 or 30 frames per second) in an aforementioned thorough way. This is what StreamDiffusion is doing, although not in a feedback loop. There have been remarkable experiments using this pipeline, but they are limited to using it as a filter, and do not benefit from potential emergent properties and consistency that you get from a feedback loop. This is only a minor (temporary) hurdle though, as proven by relentless effort of individuals such as Dan Wood (figure below), who has been able make use of the best optimization techniques in feedback loops, allowing to observe and pilot the FBF dream process as it is happening live.
EndlessDreams: Voice directed real-time video at 1280x1024. A 2+ min video gen'ed directed by my voice in 2min. A crude first start. Don't confuse smooth 60 sec vids that take hours to do. This is RT exploration of gems hidden in the latent space. This is only the beginning. pic.twitter.com/nI6akkXJhd
— Dan Wood (@Dan50412374) April 20, 2024
There can also be non-thorough approaches to real time FBF, as demonstrated by my own experiment:
Such method alleviates the pressure to generate frames fast enough through SD, allowing arbitrary gaps between them. It does however require clever techniques to blend and interpolate between the generated frames on the fly, if you care to make it appear smooth.